
By providing a set of useful features, analysts can understand trends and make tactical decisions. Features such as self-service, predictive analytics, and advanced SQL modes make these solutions easily adjustable to every level of knowledge, without the need for heavy IT involvement. Specializing in business analytics, these tools will prove to be beneficial for every data analyst that needs to analyze, monitor, and report on important findings. Business intelligence toolsīI tools are one of the most represented means of performing data analysis. Let’s get started with business intelligence tools. Like this, they get more time to perform the analytical part of their job. These data analysis tools are mostly focused on making analyst’s life’s easier by providing them with solutions that make complex analytical tasks more efficient. The image above provides a visual summary of all the areas and tools that will be covered in this insightful post.
WHAT ARE THE TOOLS FOR DATA ANALYSIS IN RESEARCH SOFTWARE
To make the most out of the infinite number of software that is currently offered on the market, we will focus on the most prominent tools needed to be an expert data analyst.

In order to make the best possible decision on which software you need to choose as an analyst, we have compiled a list of the top data analyst tools that have various focus and features, organized in software categories and represented with an example of each. 1) What Are Data Analyst Tools?ĭata analyst tools is a term used to describe software and applications that data analysts use in order to develop and perform analytical processes that help companies to make better, informed business decisions while decreasing costs and increasing profits. But first, we will start with a basic definition and a brief introduction. That said, in this article, we will cover the best data analyst tools and name the key features of each based on various types of analysis processes. Although there are many of these solutions on the market, data analysts must choose wisely in order to benefit their analytical efforts. To be able to perform data analysis at the highest level possible, analysts and data professionals will use tools and software that will ensure the best results in several tasks from executing algorithms, preparing data, generate predictions, automate processes, to standard tasks such as visualizing and reporting on the data. All other editors declare no conflicts of interest.2) The best 14 data analyst tools for 2022 Ornella Cominetti is an employee of Societé des Produits Nestlé SA. Machine/deep learning tools for analysis.Studies encompassing advances in multi-omics techniques including Metabolomics, Proteomics, Lipidomics, Genomics, Epigenomics, Transcriptomics and Metagenomics.This Research Topic welcomes Original Research, Reviews, and Reports focusing on the, but not limited to, the following subjects: Additionally, the presentation of new resources for multi-omics data analysis, such as test datasets, evaluation matrices, data standards, visualization tools, apps, packages, and others would also be a great contribution to the growing research community. The aim of this Research Topic is to showcase novel techniques and tools in the evolving field of multi-omics data analysis, in particular those focused on metabolomics data and metabolic profiling. And to reach this promise, methods and tools hold a key to make the most of the acquired data. In the context of single-cell data analysis, the analysis of several omics has made huge progress and it holds the promise to help to move further our understanding of fundamental biology and health. In the context of personalized medicine, multi-omics is playing a leading role in the identification of potential novel biomarkers.
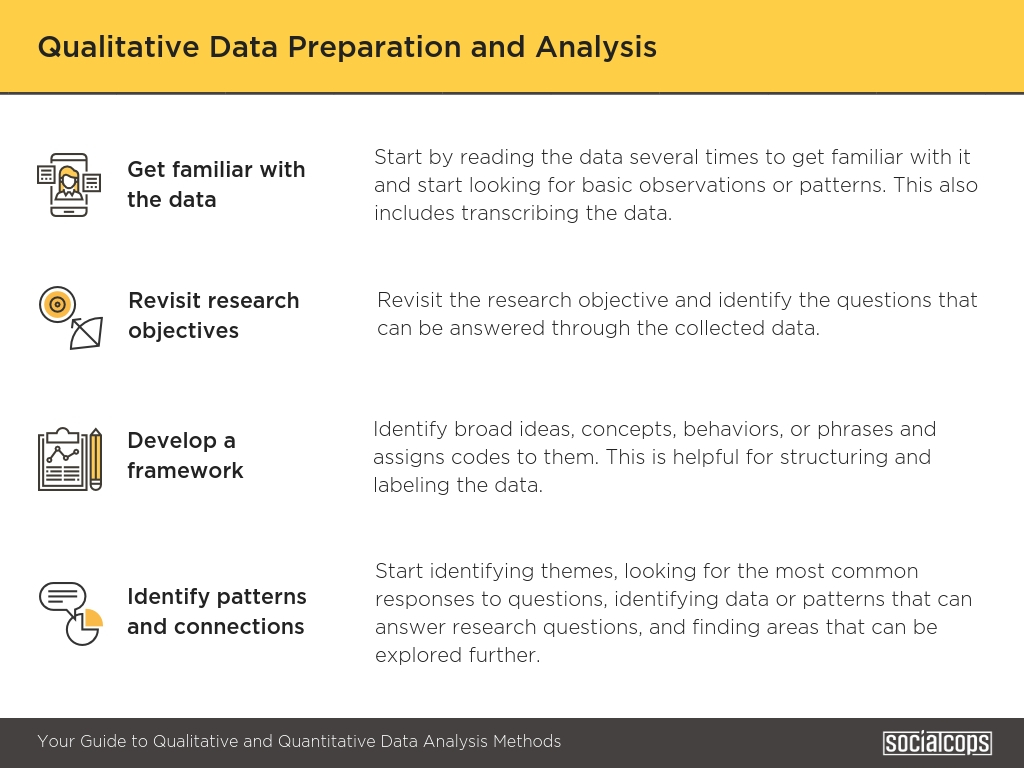
Additionally, methods are needed that are well-adapted and tested for longitudinal multi-omics datasets.
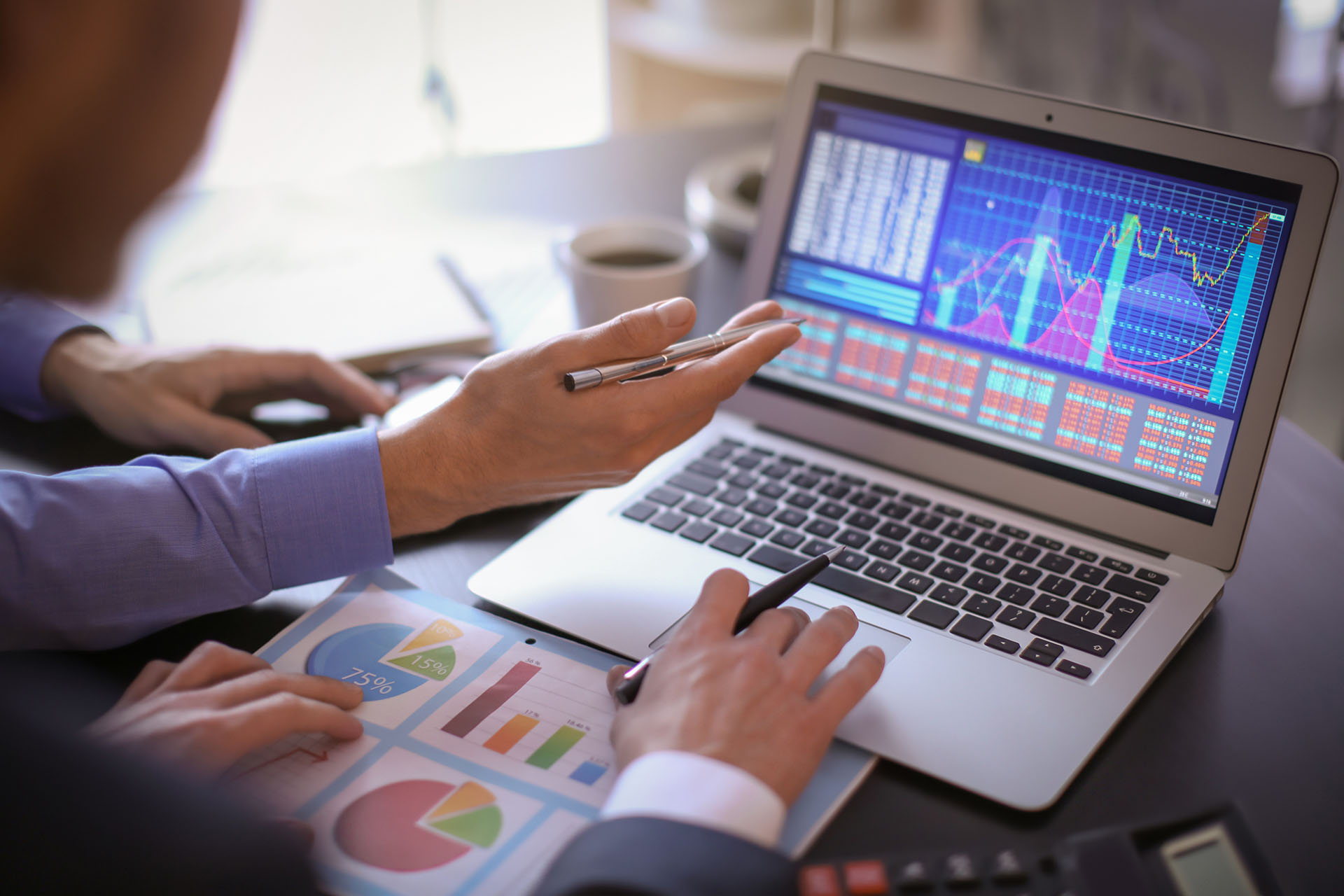
On one side, there is still a need to improve the evaluation of novel tools for multi-omics analysis. However, there are still many specific aspects to address and improve, such as standardization of the methods, a limited number of samples in most studies, and limitations on the interpretation of results, among many others. Multi-omics data analysis, also known as integrative, trans- or pan- omics is a trending and very challenging topic which has shown great advances in the last decade.
